The Role of Big Data in Autonomous Vehicles: A Look into the Future
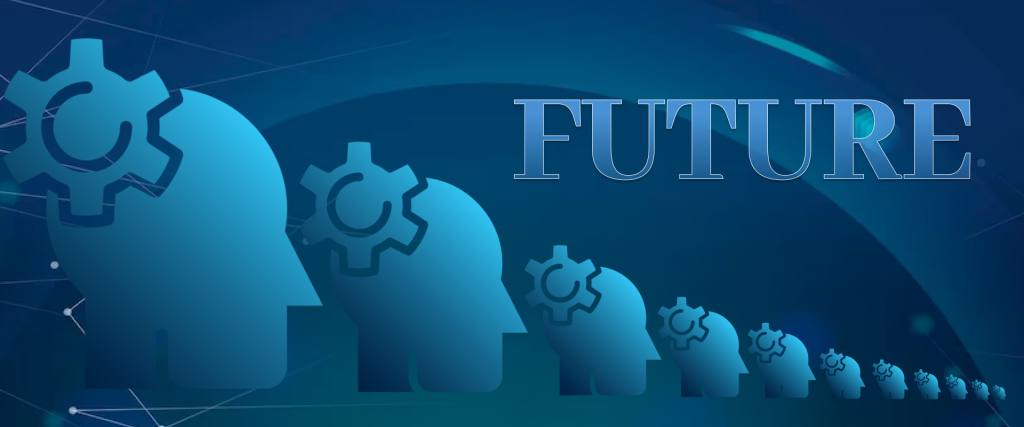
Data science has arisen as one of the fastest-growing and most in-demand fields in recent years, and its future is incredibly bright. In this blog post, we will explore some of the trends and developments that are shaping the future.
Artificial Intelligence (AI) and Machine Learning (ML)
One of the most exciting trends in data science is the integration of AI and ML technologies. In today’s Artificial Intelligence (AI) and Machine Learning (ML) are playing a important role in shaping the future of data science. As these technologies become more advanced, data scientists are able to create more smart prediction models and automate decision-making processes. This is particularly useful in industries such as finance, healthcare, and logistics, where real-time decision-making can make a significant impact.
One of the key benefits of AI and ML in data science is their ability to learn and adapt over time. Machine learning algorithms can identify patterns and insights in large datasets that may not be immediately apparent to humans, and then use this information to make predictions or guide decision-making. This has significant implications for industries such as finance, where automated trading algorithms can make trades based on real-time data and insights.
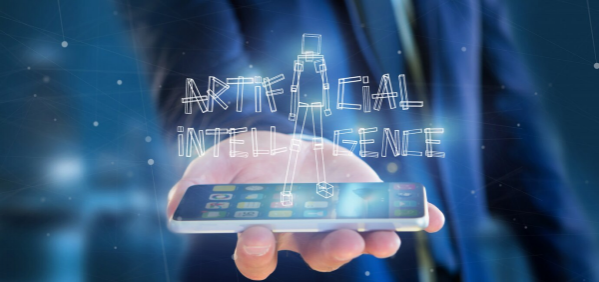
Internet of Things (IoT)
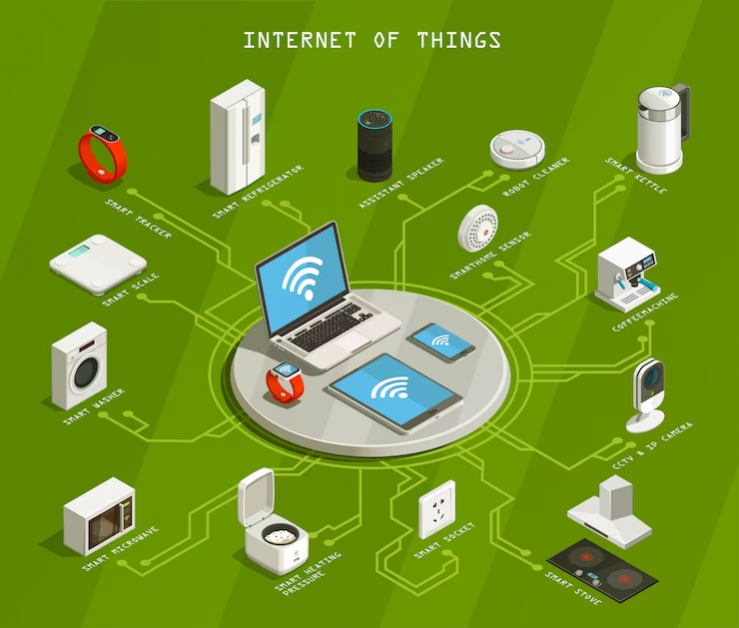
The Internet of Things (IoT) is rapidly transforming the field of data science, and its impact is only set to grow in the future. The growth of connected devices and sensors is generating massive amounts of data, which can be used to inform decision-making and create new business opportunities. Data scientists are finding new ways to extract insights from this data and use it to optimize operations and drive innovation.
IoT refers to the network of physical devices, vehicles, buildings, and other objects that are embedded with sensors, software, and connectivity that enables them to collect and exchange data.
One of the key ways in which IoT is shaping the future of data science is by generating vast amounts of data. The sensors embedded in IoT devices can collect data on everything from temperature, humidity, and air quality to traffic patterns and energy consumption. This data can then be analyzed by data scientists to gain insights into how these devices are being used, how they are performing, and how they can be optimized for greater efficiency and effectiveness.
The analysis of IoT data can also help organizations to make data-driven decisions in real-time. For example, a smart city can use IoT sensors to monitor traffic patterns and adjust traffic lights accordingly to reduce congestion. A manufacturing plant can use IoT sensors to monitor equipment performance and predict when maintenance is required, reducing downtime and increasing productivity.
Cloud Computing
Cloud computing is playing a significant role in shaping the future of data science. Cloud computing refers to the delivery of computing services, including servers, storage, databases, and software, over the internet, which enables users to access these resources from anywhere and at any time. And data science platforms and tools are enabling data scientists to scale up their operations and access powerful computing resources without the need for large IT infrastructures. This allows for greater collaboration and innovation, as data scientists can work together on large datasets and share insights across teams and organizations.
One of the key benefits of cloud computing for data science is that it provides access to vast amounts of computing power and storage capacity, which can be used to process and analyze large datasets quickly and efficiently. This has enabled data scientists to undertake more complex and sophisticated analyses than was previously possible, which has opened up new opportunities for innovation and growth.
Cloud computing also enables data scientists to collaborate and share data more easily, which can accelerate the pace of innovation and discovery. Cloud-based collaboration tools enable data scientists to work together in real-time, regardless of their location or time zone, and to share data and insights easily and securely.
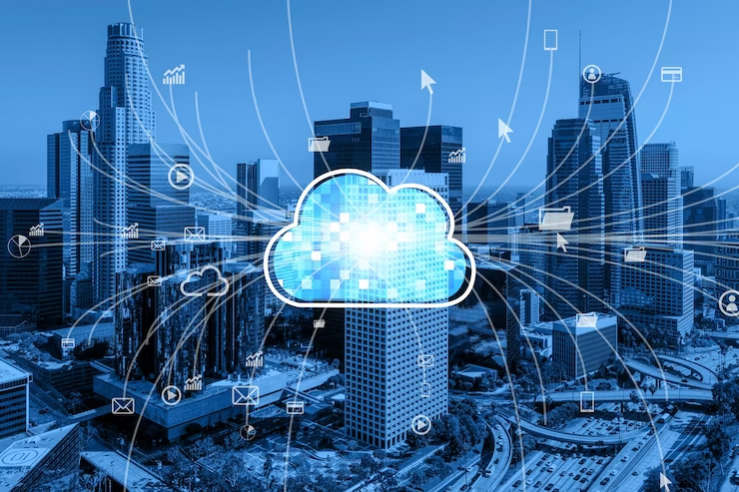
Data Privacy and Security
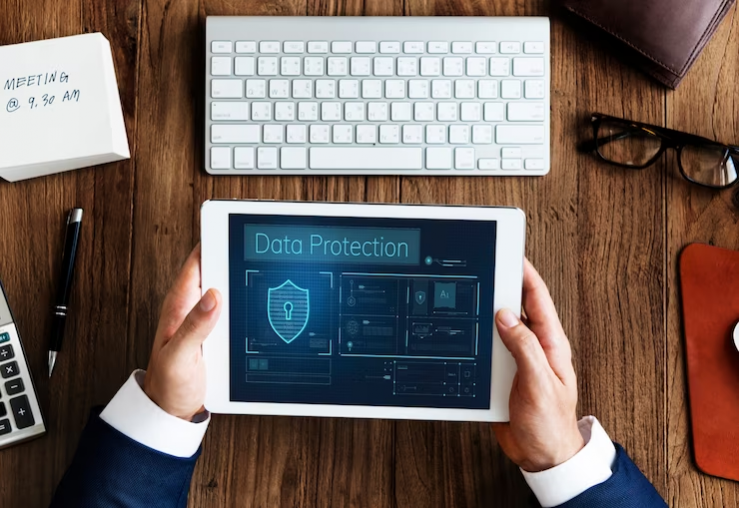
One of the ways in which data privacy and security are shaping the future of data science is by driving innovation in data protection technologies. These technologies include data encryption, access controls, and other security measures that can help to protect sensitive data from unauthorized access, theft, or misuse.
Data privacy and security are becoming increasingly important considerations in the field of data science, and are shaping its future in several ways. With the increasing volume and variety of data being collected and analyzed, data privacy and security are key concerns for individuals, organizations, and governments.
Moreover, the need for data privacy and security is driving the adoption of new technologies and techniques for data analysis, such as differential privacy, which allows organizations to analyze data while protecting individual privacy. Differential privacy provides a way to analyze data while preserving the privacy of individual data subjects by adding noise to the data before analysis.
Ethical Considerations
As data science becomes more widespread, there is a growing awareness of the ethical implications of its use. Data scientists are being called upon to ensure that their work is conducted in a responsible and transparent manner. This includes taking steps to prevent bias in algorithms, protecting user privacy, and using data in ways that benefit society as a whole.
Ethical considerations are becoming increasingly important in shaping the future of data science. With the increasing use of data science in various industries, there is a growing recognition of the need for ethical guidelines and standards to guide its use.
One of the ways in which ethical considerations are shaping the future of data science is by driving innovation in data collection and analysis techniques. Ethical considerations require that data be collected and used in a responsible and transparent manner, and that data be analyzed in a way that does not discriminate or harm individuals or groups. This has led to the development of techniques such as differential privacy, which allow data to be analyzed while preserving the privacy of individual data subjects.
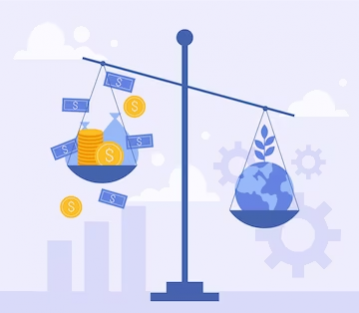
Conclusion
In conclusion, the future of data science is incredibly bright, with exciting opportunities for innovation and growth. As data becomes more ubiquitous and valuable, data scientists will continue to play a critical role in helping organizations to make better decisions, solve complex problems, and create new business opportunities. However, as data science continues to evolve, it is important that data scientists prioritize ethical considerations and ensure that they are using data in ways that benefit society as a whole.